How Visit-Level Attribution Makes Marketing Attribution Predictive Instead of Purely Retrospective
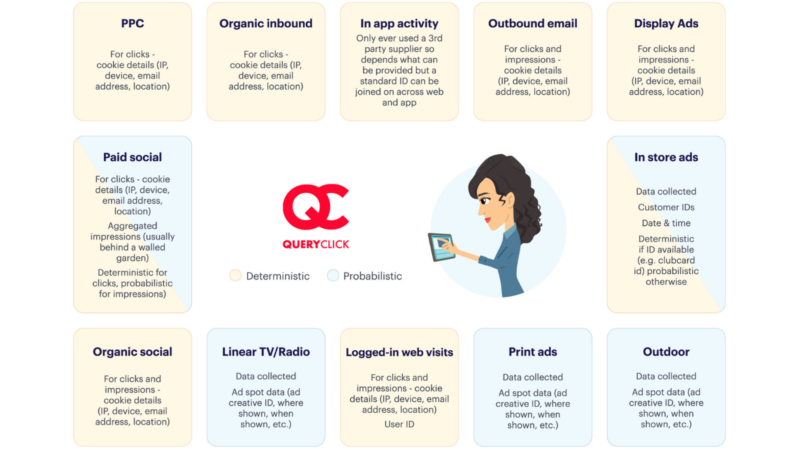
Today, one thing marketers can’t complain about is the amount of data they receive as a result of their advertising and other promotional activities.
A typical marketing communications mix will include PPC advertising, e-mail blasts, blogs, and other content marketing, PR, offline advertising on linear TV and radio, in-store promotions, social media, and perhaps even outdoor billboards and sponsorship. It can sometimes seem that there’s a new ‘must-have’ channel every month but the data that comes from many of these channels is siloed and difficult to interpret.
In fact, according to eMarketer’s Global Digital Marketing Report and Statista, siloed marketing data will have increased by 32x between 2011 and 2021.
But the sheer volume of data is causing more problems than it’s solving.
Despite a plethora of software companies promising marketing attribution platforms that will show marketers where to best target their advertising and other promotional spend, attribution attempts have been dogged by inaccuracies and a lack of trust has set in.
With the worldwide digital ad spend at $333.25 billion in 2019, with roughly the same amount allocated to traditional advertising, there is an urgent need for attribution to be improved.
Billions of dollars of advertising investment will be wasted if it’s not.
What progress has been made in marketing attribution?
Many attribution models have evolved as attempts to improve on the ‘last-click’ attribution model so beloved by Google Analytics.
Last-click attribution still drives most budget allocation decisions, even though Google’s Digital Marketing Evangelist, Avinash Kaushik, said in this blog post from 2013: “The only use for last-click attribution now is to get you fired. Avoid it.” Kaushik goes on to describe in detail several alternative models and how to explore them.
The challenge with all of them is that the weightings given to the various touch-points – places where consumers come into contact with your brand – are guesswork. Informed guesswork perhaps, but guesswork, nevertheless.
Data that marketers receive on consumer behaviour fall into two categories: deterministic data, where you can be certain of the identity of an individual that has interacted with your brand, and probabilistic data.
The former is derived when someone is logged on to your website or has downloaded and is using your app. The latter is the kind of data you might receive from offline advertising or social media channels, like Facebook or Amazon.
You know that your paid, earned, or shared channel exposure is likely to have reached a given number of people that fit within the profile of your target consumer.
But it’s not possible to be certain that someone who takes the desired action on your website was one of those consumers.
It’s not all bad news.
Probability modelling, augmented by the use of artificial intelligence algorithms, is continuously improving the accuracy of the data we can extract from data silos.
We can have greater confidence that we understand how probable it is that we touched a target consumer within a given channel, with a particular campaign.
However, a bigger challenge has been how to stitch together probabilistic and deterministic data in a meaningful way to gain a 360-degree view of the customer journey, their touchpoints along the way, and the likely impact of each touchpoint on the customers’ decision to engage with a brand by visiting the marketer’s website, sharing their email address or making a purchase.
There have been significant advances here too.
The most advanced tools now cleaning up ‘dirty’ and inaccurate data so that it is normalised and restructured before it’s joined with sessions from other silos.
Only then is it analysed using machine learning to deliver over three times more reliable attribution than the over-simplistic, rules-based approaches described by Kaushik and others.
But even with this level of analytical sophistication, marketers are limited to reacting to what customers have done, rather than being able to predict what they’re likely to do next when they encounter a brand.
VLA takes attribution to the predictive level with the help of neural networks
‘Visit-Level Attribution’ or VLA, is a new process based on neural networks, a branch of artificial intelligence that mimics how the human brain works.
Developed in partnership with the University of Edinburgh, this technique analyses how customers behave when they encounter marketing collateral, wherever they see it or interact with it, online or offline.
The resulting data is far more granular than that which comes from simply measuring impressions and clicks.
Using VLA, over 300 characteristics of customer engagements with marketing collateral are mapped to predict what the next level of engagement will be. The technique unbundles siloed data from channels such as Facebook and Google.
And because it only analyses first-party pixel data that complies with GDPR personal consent requirements, it’s beneficial to advertisers who are concerned with compliance and to consumers who have privacy worries. It’s a significant step forward for data privacy.
VLA gives marketers an accurate view of an individual’s probability to convert.
Online data can be laid alongside offline data to identify overlaps in time, location, and other identifiers.
When a customer engages at a different rate than expected after, for example, seeing an advertisement, that advertisement is stitched into the journey for attribution.
Another benefit of VLA is eliminating the commonplace need for marketers to rely on second-party relationships to obtain data, much of which is not GDPR compliant.
Predicting human behaviour is always going to be difficult but the closer marketers can get to doing so, at least when it comes to customers’ buying journeys, the more money they will save and the more effective their efforts will be.
And it’s a multi-billion-dollar global challenge. Visit-Level-Attribution is an important step in the marketing journey towards a better understanding of customer intent and behaviour.
For more detailed information on marketing attribution technology and its capabilities, download ‘The Complete Guide to Marketing Attribution’.
What is Visit Level Attribution?
Own your marketing data & simplify your tech stack.
Have you read?
I have worked in SEO for 12+ years and I’ve seen the landscape shift a dozen times over. But the rollout of generative search engines (GSEs) feels like the biggest...
As you will have likely seen, last week Google released the March 2024 Core Algorithm Update. With it, comes a host of changes aiming to improve the quality of ranking...
After a year of seemingly constant Google core updates and the increasingly widespread usage of AI, the SEO landscape is changing more quickly than ever. With this rapid pace of...